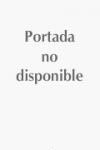
Internet of Things and Machine Learning for Type I and Type II Diabetes: Use cases
Dash, Sujata
Pani, Subhendu Kumar
Susilo, Willy
Yung Bernard, Cheung Man
Tse, Gary
Internet of Things and Machine Learning for?Type I and Type II Diabetes: Use Cases provides a medium of exchange of expertise and addresses the concerns, needs, and problems associated with Type I and Type II diabetes. Expert contributions come from researchers across biomedical, data mining, and deep learning. This is an essential resource for both the AI and Biomedical research community, crossing various sectors for broad coverage of the concepts, themes, and instrumentalities of this important and evolving area. Coverage includes IoT, AI, Deep Learning, Machine Learning and Big Data Analytics for diabetes and health informatics. Integrates many Machine learning techniques in biomedical domain to detect various types of diabetes to utilizing large volumes of available diabetes-related data for extracting knowledgeIt integrates data mining and IoT techniques to monitor diabetes patients using their medical records (HER) and administrative dataIncludes clinical applications to highlight contemporary use of these machine learning algorithms and artificial intelligence-driven models beyond research settings INDICE: Section 1: Diagnosis 1. An Intelligent Diagnostic approach for diabetes Using rule-based Machine Learning techniques 2. Ensemble Sparse Intelligent Mining Techniques for Diabetes Diagnosis 3. Detection of Diabetic Retinopathy Using Neural Networks 4. An Intelligent Remote Diagnostic Approach for Diabetes Using Machine Learning Techniques 5. Diagnosis of Diabetic Retinopathy in Retinal Fundus Images Using Machine Learning and Deep Learning Models 6. Diagnosis of Diabetes Mellitus using Deep Learning Techniques and Big Data Section 2: Glucose monitoring 7. IoT and Machine Learning for Management of Diabetes Mellitus 8. Prediction of glucose concentration in type 1 diabetes patients based on Machine learning techniques 9. ML-Based PCA Methods to Diagnose Statistical Distribution of Blood Glucose Levels of Diabetic Patients Section 3: Prediction of complications and risk stratification 10. Overview of New trends on deep learning models for diabetes risk prediction 11. Clinical applications of deep learning in diabetes and its enhancements with future predictions 12. Feature Classification and Extraction of Medical Data Related to Diabetes Using Machine Learning Techniques: A Review 13. ML-based predictive model for type 2 diabetes mellitus using genetic and clinical data 14. Applications of IoT and data mining techniques for diabetes monitoring 15. Decision-making System for the Prediction of Type II Diabetes Using Data Balancing and Machine Learning Techniques 16. Comparative Analysis of Machine Learning Tools in Diabetes Prediction 17. Data Analytic models of patients dependent on insulin treatment 18. Prediction of Diabetes using Hybridization of Radial Basis Function Network and Differential Evaluation based Optimization Technique 19. An Overview of New Trends On Deep Learning Models For Diabetes Risk Prediction Section 4: Dialysis 20. Progression and Identification of heart disease risk factors in diabetic patients from electronic health records 21. An Intelligent Fog Computing-based Diabetes Prediction System for Remote Healthcare Applications 22. Artificial intelligence approaches for risk stratification of diabetic kidney disease 23. Computational Methods for predicting the occurrence of cardiac autonomic neuropathy 24. Development of a Clinical Forecasting Model to Predict Comorbid Depression in Diabetes Patients and its Application in Policy Making for Depression Screening Section 5: Drug design and Treatment Response 25. Enhancing Diabetic Maculopathy Classification through a Synergistic Deep Learning Approach by Combining Convolutional Neural Networks, Transfer Learning, and Attention Mechanisms 26. Pharmacogenomics: the roles of genetic factors on treatment response and outcomes in diabetes 27. Predicting treatment response in diabetes: the roles of machine learning-based models 28. Antidiabetic Potential of Mangrove Plants: An Updated Review
- ISBN: 978-0-323-95686-4
- Editorial: Elsevier
- Encuadernacion: Rústica
- Páginas: 500
- Fecha Publicación: 15/07/2024
- Nº Volúmenes: 1
- Idioma: Inglés