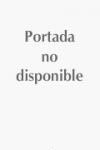
Design and analysis of learning classifier systems: a probabilistic approach
Drugowitsch, J.
This book provides a comprehensive introduction to the design and analysis ofLearning Classifier Systems (LCS) from the perspective of machine learning. LCS are a family of methods for handling unsupervised learning, supervised learning and sequential decision tasks by decomposing larger problem spaces into easy-to-handle subproblems. Contrary to commonly approaching their design and analysis from the viewpoint of evolutionary computation, this book instead promotes a probabilistic model-based approach, based on their defining question ‘What is an LCS supposed to learn?’. Systematically following this approach, it is shown how generic machine learning methods can be applied to design LCS algorithms from the first principles of their underlying probabilistic model, which is in this book – for illustrative purposes – closely related to the currently prominent XCS classifier system. Latest research in the area of Learning Classifier Systems Presents a probabilistic approach to Design and Analysis of Learning Classifier Systems INDICE: Introduction.- Background.- A Learning Classifier Systems Model.- A Probabilistic Model for LCS.- Training the Classifiers.- Mixing Independently Trained Classifiers.- The Optimal Set of Classifiers.- An Algorithmic Description.- Towards Reinforcement Learning with LCS.- Concluding Remarks.
- ISBN: 978-3-540-79865-1
- Editorial: Springer
- Encuadernacion: Cartoné
- Páginas: 268
- Fecha Publicación: 01/07/2008
- Nº Volúmenes: 1
- Idioma: Inglés