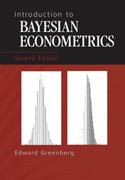
This textbook explains the basic ideas of subjective probability and shows how subjective probabilities must obey the usual rules of probability to ensure coherency. It defines the likelihood function, prior distributions and posterior distributions. It explains how posterior distributions are the basis for inference and explores their basic properties. Various methods of specifying prior distributions are considered, with special emphasis on subject-matter considerations and exchange ability. The regression model is examined to show how analytical methods may fail in the derivation of marginal posterior distributions. The remainder of the book is concerned with applications of the theory to important models that are used in economics, political science, biostatistics and other applied fields. New to the second edition is a chapter on semiparametric regression and new sections on the ordinal probit, item response, factor analysis, ARCH-GARCH and stochastic volatility models. The new edition also emphasizes the R programming language. INDICE: Part I. Fundamentals of Bayesian Inference: 1. Introduction; 2. Basic concepts of probability and inference; 3. Posterior distributions and inference; 4. Prior distributions; Part II. Simulation: 5. Classical simulation; 6. Basics of Markov chains; 7. Simulation by MCMC methods; Part III. Applications: 8. Linear regression and extensions; 9. Semiparametric regression; 10. Multivariate responses; 11. Time series; 12. Endogenous covariates and sample selection; A. Probability distributions and matrix theorems; B. Computer programs for MCMC calculations.
- ISBN: 978-1-107-01531-9
- Editorial: Cambridge University Press
- Encuadernacion: Cartoné
- Páginas: 264
- Fecha Publicación: 12/11/2012
- Nº Volúmenes: 1
- Idioma: Inglés