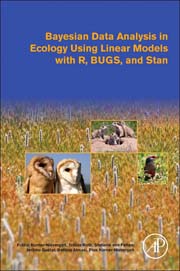
Bayesian Data Analysis in Ecology Using Linear Models with R, BUGS, and STAN: Including Comparisons to Frequentist Statistics
Korner-Nievergelt, Franzi
von Felten, Stefanie
Roth, Tobias
Almasi, Bettina
Guélat, Jérôme
Korner-Nievergelt, Pius
Bayesian Data Analysis in Ecology Using Linear Models with R, BUGS, and STAN introduces the Bayesian and frequentist methods of conducting data analyses. The book provides the theoretical background in an easy to understand way and it encourages the reader to think about the processes that generated their data. Model selection and multi-model inference are discussed and effort is made to create effect plots that allow a much more natural interpretation of the data rather than simple parameter estimates. Model checking by graphical analysis and by posterior predictive checking is also discussed. Bayesian Data Analysis in Ecology Using Linear Models with R, BUGS, and STAN encourages readers to think about the processes that generated their data and to build models that reflect these processes as close as possible. Therefore, the Bayesian software, BUGS, JAGS, STAN and LaplacesDemon are introduced. This book guides the reader from easy towards more complex (real) data analyses, step by step. The problems and solutions, including all R codes, presented in the book are most often replicable to other data and questions. Thus, this book can be used continually as a resource for all sorts of questions and field data collected. Offers Bayesian data analysis, allowing users to obtain uncertainty measurements easily for any derived parameter of interestWritten in a step-by-step approach, which is accessible to non-statisticiansIncludes companion website containing R-code to help users conduct Bayesian data analyses on their own data INDICE: 1. Why do we need statistical models2. Prerequisites and vocabulary3. The Bayesian and frequentist way of analyzing data4. Normal linear model LM5. Likelihood6. Assessing model assumptions7. Linear mixed effects model LMM8. Generalized linear model GLM9. Generalized linear mixed model GLMM10. Posterior predictive model checking and proportion of explained variance11. Model selection and multi-model inference12. Markov chain Monte Carlo simulation (MCMC)13. Modeling spatial data using GLMM14. Advanced ecological models15. Prior influence and parameter estimability16. Checklist17. What to reportAcknowledgmentsReferences
- ISBN: 978-0-12-801370-0
- Editorial: Academic Press
- Encuadernacion: Rústica
- Páginas: 256
- Fecha Publicación: 27/05/2015
- Nº Volúmenes: 1
- Idioma: Inglés