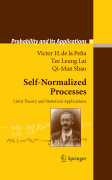
Self-normalized processes: limit theory and statistical applications
Peña, V.H.
Lai, T.L.
Shao, Q.
Self-normalized processes are of common occurrence in probabilistic and statistical studies. A prototypical example is Student's t-statistic introduced in 1908 by Gosset, whose portrait is on the front cover. Due to the highly non-linear nature of these processes, the theory experienced a long period of slow development. In recent years there have been a number of important advances in the theory and applications of self-normalized processes. Some of these developments are closely linked to the study of central limit theorems, which imply that self-normalized processes are approximate pivots for statistical inference. The present volume covers recent developments in the area, including self-normalized large and moderate deviations, and laws of the iterated logarithms for self-normalized martingales. This is the first book that systematically treats the theory and applications of self-normalization. First book that systematically treats the theory and applications of Self-Normalization Fills a current void in PhD level courses in probability and statistics offered by major Statistics departments Rich enough in its coverage to provide such a second course for PhD students Integrates advanced probability with theoretical statistics, instead of presenting them as two disparate subjects INDICE: From the contents Part I Independent Random Variables.- 2. Classical Limit Theorems and Preliminary Tools.- 3. Self-Normalized Large Deviations.- 4. Weak Convergence of Self-Normalized Sums.- 5. Stein’s Method and Self-Normalized Berry–Esseen Inequality.- 6. Self-Normalized Moderate Deviations and Law of the Iterated Logarithm.- 7. Cramér-type Moderate Deviations for Self-Normalized Sums.- 8. Self-Normalized Empirical Processes and U-Statistics.- Part II Martingales and Dependent Random Vectors.- 9. Martingale Inequalities and Related Tools.- 10. A General Framework for Self-Normalization.- 11. Pseudo-Maximization via Method of Mixtures.- 12. Moment and Exponential Inequalities forSelf-Normalized Processes.- 13. Laws of the Iterated Logarithm for Self-Normalized Processes and Martingales.- 14. Multivariate Matrix-Normalized Processes.- Part III Statistical Applications.- 15. The t-Statistic and Studentized Statistics.
- ISBN: 978-3-540-85635-1
- Editorial: Springer
- Encuadernacion: Cartoné
- Páginas: 260
- Fecha Publicación: 01/10/2008
- Nº Volúmenes: 1
- Idioma: Inglés