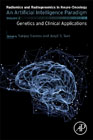
Radiomics and Radiogenomics in Neuro-Oncology: An Artificial Intelligence Paradigm - Volume 2: Genetics and Clinical Applications
Saxena, Sanjay
Suri, Jasjit
Neuro-oncology broadly encompasses life-threatening brain and spinal cord malignancies, including primary lesions and lesions metastasizing to the central nervous system. It is well suited for diagnosis, classification, and prognosis as well as assessing treatment response. Radiomics and Radiogenomics (R-n-R) have become two central pillars in precision medicine for neuro-oncology. Radiomics is an approach to medical imaging used to extract many quantitative imaging features using different data characterization algorithms, while Radiogenomics, which has recently emerged as a novel mechanism in neuro-oncology research, focuses on the relationship of imaging phenotype and genetics of cancer. Due to the exponential progress of different computational algorithms, AI methods are composed to advance the precision of diagnostic and therapeutic approaches in neuro-oncology. The field of radiomics has been and definitely will remain at the lead of this emerging discipline due to its efficiency in the field of neuro-oncology. Several AI approaches applied to conventional and advanced medical imaging data from the perspective of radiomics are very efficient for tasks such as survival prediction, heterogeneity analysis of cancer, pseudo progression analysis, and infiltrating tumors. Radiogenomics advances our understanding and knowledge of cancer biology, letting noninvasive sampling of the molecular atmosphere with high spatial resolution along with a systems-level understanding of causal heterogeneous molecular and cellular processes. These AI-based R-n-R tools have the potential to stratify patients into more precise initial diagnostic and therapeutic pathways and permit better dynamic treatment monitoring in this period of personalized medicine. While extremely promising, the clinical acceptance of R-n-R methods and approaches will primarily hinge on their resilience to non-standardization across imaging protocols and their capability to show reproducibility across large multi-institutional cohorts. Radiomics and Radiogenomics in Neuro-Oncology: An Artificial Intelligence Paradigm - Volume 2: Genetics and Clinical Applications provides readers with a broad and detailed framework for R-n-R approaches with AI in neuro-oncology, the description of cancer biology and genomics study of cancer, and the methods usually implemented for analyzing. Readers will also learn about the current solutions R-n-R can offer for personalized treatments of patients, limitations, and prospects. There is comprehensive coverage of information based on radiomics, radiogenomics, cancer biology, and medical image analysis viewpoints on neuro-oncology, so this in-depth coverage is divided into two Volumes. Volume 1: Radiogenomics Flow Using Artificial Intelligence provides coverage of genomics and molecular study of brain cancer, medical imaging modalities and analysis in neuro-oncology, and prognostic and predictive models using radiomics. Volume 2: Genetics and Clinical Applications provides coverage of imaging signatures for brain cancer molecular characteristics, clinical applications of R-n-R in neuro-oncology, and Machine Learning and Deep Learning AI approaches for R-n-R in neuro-oncology. Includes coverage of foundational concepts of the emerging fields of Radiomics and Radiogenomics, developing the Neural Engineering modelling and AI algorithms for imaging, diagnosis and predictive modeling of neuro-oncologyCovers imaging signatures for brain cancer molecular characteristics, including Isocitrate Dehydrogenase Mutations (IDH), TP53 Mutations, ATRX loss, MGMT gene, Epidermal Growth Factor Receptor (EGFR), and other mutationsPresents clinical applications of R-n-R in neuro-oncology such as risk stratification, survival prediction, heterogeneity analysis, as well as early and accurate prognosisProvides in-depth technical coverage of radiogenomics studies for difference brain cancer types, including glioblastoma, astrocytoma, CNS lymphoma, meningioma, acoustic neuroma, and haemangioblastoma INDICE: Section 1: Imaging signatures for brain cancer molecular characteristics 1. Isocitrate Dehydrogenase Mutations (IDH) 2. TP53 Mutations 3. ATRX Loss 4. MGMT (O6-Methylguanine-DNA-Methyltransferase Methylation) gene 5. EGFR (Epidermal Growth Factor Receptor) 6. Other mutations Section 2: Clinical applications of R-n-R in Neuro-Oncology 7. Risk Stratification 8. Survival Prediction 9. Heterogeneity Analysis 10: Early and Accurate Prognosis Section 3: Radiogenomics studies for different brain cancer types 11. Glioblastoma 12. Astrocytoma 13. CNS lymphoma 14. Others brain cancers: Meningioma, Acoustic neuroma, Haemangioblastoma Section 4: AI in R-n-R for Neuro-Oncology: What we have achieved so Far? 15. A Survey on recent advancement of AI-enabled R-n-R in neuro-oncology 16. Prospects and advances in R-n-R 17. Progress and future aspects 18. Limitations of AI in R-n-R study
- ISBN: 978-0-443-18509-0
- Editorial: Academic Press
- Encuadernacion: Rústica
- Páginas: 622
- Fecha Publicación: 01/11/2024
- Nº Volúmenes: 1
- Idioma: Inglés